Alzheimer’s disease (AD), a progressive incurable neurodegenerative disorder characterised by memory loss and functional decline, is a serious increasing public health problem.[1] It is predicted to strain global health care systems in the future.[2]
In the pharmaceutical industry, substantial resources have been expended over recent decades to identify treatments that may slow the natural history of the disease, especially during the precursor stage of mild cognitive impairment (MCI).[3]
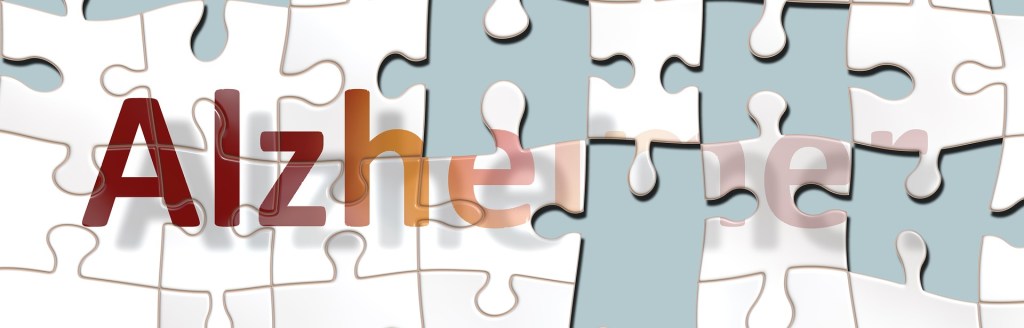
Common clinical trials include patients with AD and/or MCI with or without other health conditions, such as Parkinson’s disease, depression and sleep disorders (Table 1). But, to date, these efforts have delivered a small number of modestly efficacious treatments.[4] For example, the success rates for drug agents advancing to FDA regulatory approval for market has been estimated to be 0.4%.[5]
Reasons for low success rates in MCI/AD drug development include failure to develop new compounds that demonstrate efficacy and trial methodological issues including appropriateness of patient centered outcome (PCO) measures.[6][7]
Did you know…
Modus Outcomes has long standing experience researching PCO measures in MCI/AD clinical studies, spanning pharmaceutical clinical trials, [8] natural history studies, [9][10] and academic projects.[11]
Currently, Modus Outcomes is part of the European EMPIR NeuroMet 15HLT04 project (2016-2019), [12] which combines expertise from national metrology, clinical and academic institutions.
The goal of the NeuroMet project is to address the limitations currently constraining clinical innovation and uptake in neurodegenerative disease diagnosis and treatment, in measurement sensitivity and high measurement variability of recognised and novel biomarkers for AD (e.g. lumbar puncture and blood sampling) as well as neuroimaging (non-invasive magnetic resonance imaging and spectroscopy).
As part of EMPIR NeuroMet 15HLT04 project, PCO measures of cognitive performance are examined, with the ultimate goal of improving the analysis of correlations with the various MCI/AD biomarkers. These measures usually include tasks related to recall, language and praxis. A range of these kinds of PCO measures have been used as primary and secondary endpoints in pharmaceutical clinical trials in recent years (Table 2).
Prospective data collection from a NeuroMet cohort recruited by the Charité hospital in Berlin, Germany, and psychometric analysis of that data, is now in progress to develop a ‘NeuroMet Memory Score’. This is based on results from administering an extensive battery of legacy instruments (e.g., Mini-Mental State Examination, Corsi Block Test, Digital Span Test). The resultant improved estimates across comprehensive spans of cognitive task difficulty and patient ability, furnished by the Rasch model, are in turn enabling formulation of novel construct specification equations for correlation using multivariate principal component regression with, respectively, task entropy (e.g., logarithm of number of digits in DST) and diverse biomarkers (e.g., for brain atrophy).[13]
Establishing new cognitive performance measurements should lead to more fit-for-purpose, better targeted and better administered cognitive instruments anchored in item banks of quality-assured metrological references for cognitive task difficulty and patient ability. By exploiting the potential of causal Rasch models, metrological references for cognitive task difficulty, akin to certified reference materials, promise to improve the quality of patient-centred care of MCI and AD. This will enable the traceable calibration of both additional cognitive tasks, as well as the effects of intervention or disease progression on the cognitive ability of each individual patient.
Further Reading
[1] Brookmeyer R, Johnson E, K Z-G, MH A. Forecasting the global burden of Alzheimer’s disease. Alzheimer’s & Dementia. 2007;3(3):186-191.
[2] Thies W, Bleiler L. Alzheimer’s disease facts and figures. Alzheimer’s & Dementia. 2013;9(2):208-245.
[3] Anand A, Patience A, Sharma N, Khurana N. The present and future of pharmacotherapy of Alzheimer’s disease: A comprehensive review. European Journal of Pharmacology. 2017;815:364-375.
[4] Anderson R, Hadjichrysanthou C, Evans S, Wong M. Why do so many clinical trials of therapies for Alzheimer’s disease fail? Lancet. 2017;390(10110):2327-2329.
[5] Cummings J. Alzheimer’s disease drug development pipeline: few candidates, frequent failures. Alzheimer’s Research &Therapy. 2014;6:37.
[6] Hobart J, Cano S, Zajicek J, Thompson A. Rating scales as outcome measures for clinical trials in neurology: problems, solutions, and recommendations. Lancet Neurology. 2007;6(12):1094-1105.
[7] Cummings J. Lessons Learned from Alzheimer Disease: Clinical Trials with Negative Outcomes. Clin Transl Sci. 2018;11(2):147-152.
[8] Cano S, Posner H, Moline M, et al. The ADAS-cog in Alzheimer’s Disease clinical trials: Psychometric evaluation of the sum and its parts. Journal of Neurology Neurosurgery and Psychiatry. 2010;81(12):1363-1368.
[9] Cano S, Hobart J, Posner H, et al. Putting the Alzheimer’s cognitive test to the test I: traditional psychometric methods. Alzheimer’s & Dementia. 2013;9(1 Suppl):S4-S9.
[10] Cano S, Hobart J, Posner H, et al. Putting the Alzheimer’s cognitive test to the test II: Rasch Measurement Theory. Alzheimer’s & Dementia. 2013;9(1 Suppl):S10-20.
[11] Hendriks A, Smith S, Chrysanthaki T, Cano S, Black N. DEMQOL and DEMQOL-Proxy: a Rasch analysis. Health and Quality of Life Outcomes. 2017;15:164.
[12] EMPIR. EMPIR Project 15HLT04 NeuroMet, Innovative measurements for improved diagnosis and management of neurodegenerative diseases. 2016-2019.
[13] Pendrill L. Assuring measurement quality in person-centred healthcare. Measurement Science and Technology. 2018;29(3):034003.